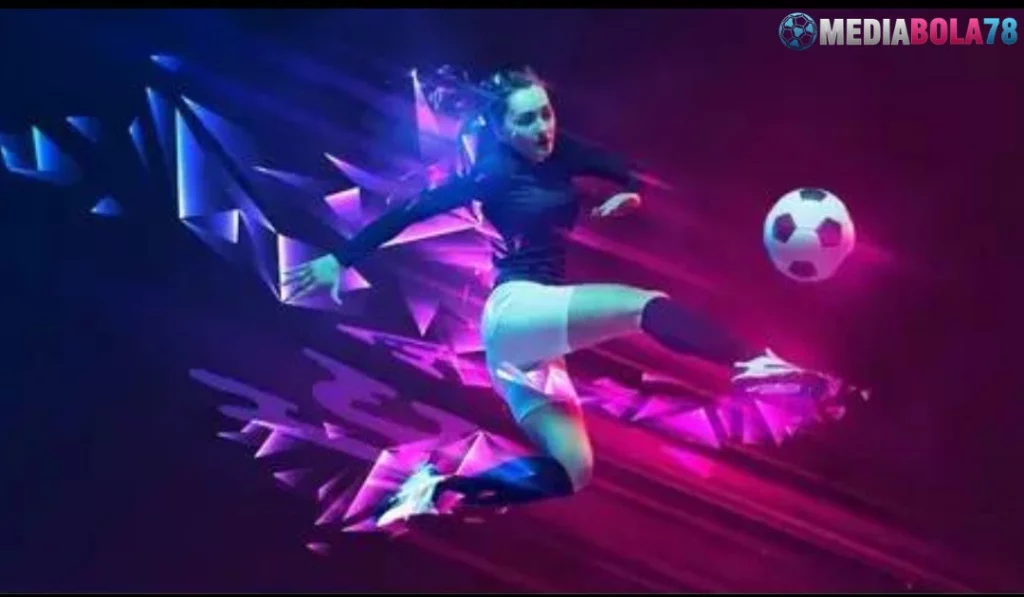
MEDIABOLA78: Revolusi Slot Online Berkualitas
Dalam dunia yang terus berkembang, terutama di sektor hiburan digital, MEDIABOLA78 telah menempatkan dirinya sebagai pusat permainan slot online, membawa angin segar dalam industri perjudian dengan serangkaian inovasi dan layanan berkualitas. Artikel ini akan membahas secara mendalam tentang bagaimana MEDIABOLA78 berhasil merevolusi pengalaman bermain slot online, melalui penggunaan teknologi modern, pilihan permainan yang luas, serta komitmen terhadap kepuasan dan keamanan pelanggan.
Penerapan Teknologi Modern
MEDIABOLA78 memahami pentingnya teknologi dalam menyediakan pengalaman bermain yang memuaskan. Dengan menggunakan platform yang dioptimalkan untuk semua perangkat, baik desktop maupun mobile, MEDIABOLA78 memastikan bahwa setiap pemain dapat mengakses permainan favorit mereka dengan mudah, kapan saja dan di mana saja. Keamanan data pengguna menjadi prioritas utama, dengan penerapan enkripsi data canggih untuk melindungi informasi pribadi dan transaksi finansial pemain.
Variasi Permainan yang Luas
Keberagaman permainan adalah salah satu kekuatan utama MEDIABOLA78. Situs ini menawarkan berbagai jenis slot online yang dirancang untuk memenuhi selera dan preferensi berbagai pemain, dari slot klasik hingga slot video modern dengan tema yang menarik dan fitur-fitur inovatif. Permainan baru secara berkala ditambahkan ke koleksi untuk menjaga agar pengalaman bermain selalu segar dan menarik.
Komitmen Terhadap Kepuasan Pelanggan
MEDIABOLA78 tidak hanya fokus pada aspek teknis dan variasi permainan, tetapi juga sangat memperhatikan kepuasan pelanggan. Dukungan pelanggan yang responsif dan profesional siap membantu pemain dengan setiap pertanyaan atau masalah yang mungkin timbul. Selain itu, situs ini juga menawarkan bonus dan promosi yang menarik, dirancang untuk memberikan nilai tambah bagi pengguna, baik pemain baru maupun yang sudah lama bergabung.
Kesimpulan
MEDIABOLA78 berhasil memposisikan dirinya sebagai pemimpin dalam industri slot online dengan menggabungkan inovasi teknologi, variasi permainan, dan komitmen terhadap kepuasan pelanggan. Melalui upaya ini, MEDIABOLA78 tidak hanya menyediakan hiburan yang berkualitas tetapi juga menciptakan lingkungan bermain yang aman dan terpercaya. Dengan terus berinovasi dan memperhatikan kebutuhan pemain, MEDIABOLA78 berjanji akan tetap menjadi pilihan utama bagi para penggemar slot online.
© Copyright 2000 – 2024 MEDIABOLA78